“Talent wins games, but teamwork and intelligence win championships.” is a famous quote by Michael Jordan. Be it the workplace or playground team efforts always lead to success. In the current AI driven landscape organizations are witnessing a new challenge of augmenting the intelligence of human resources and that of machine intelligence to maximize productivity. Yes, today’s business environment demands collaboration and coordination between not only human resources but also between human and machine intelligence. We are in the era of Collaborative Intelligence engines and this blog is an attempt to decode the intricacies of this emerging area of Artificial Intelligence.
What are Collaborative Intelligence Engines, and Why is it Important?
Consider you are working on a software project alongside your colleagues. Your development skills and your colleagues’ debugging or testing skills go hand in hand to build a robust software product—Ditto with Human and AI collaboration. Collaborative Intelligence engines foster an environment where Humans work alongside “AI tools” enhancing each other’s strengths. Often proponents of collaborative intelligence classify the activities at the workplace under two broad categories: Human Assisting Machines (AI) and Machines Assisting Humans.
With collaborative intelligence, humans can focus on pursuing higher-order thinking capabilities like creativity and building positive human connections and AI agents can work on tasks like enhancing scalability and automate repetitive tasks.
A Three-Step Approach to Identify Collaborative Intelligence
The industry has arrived at Collaborative Intelligence as the third step by going through two prior steps of business transformation.
The first step was witnessed through standardization of all the manufacturing processes like in an assembly line.
The second step gained momentum with the advent of the PC and the Internet. Automation of all the activities with systems like Robotic Process Automation (RPA) as a key feature of this step.
The third step is what we can identify as Collaborative Intelligence where humans and machines work in tandem to reap the strengths of both players.
The Collaborative Intelligence Engines Playground and Players
Organizations are quickly understanding that AI capabilities are not meant to replace human resources, in fact, they prove to enhance workplace productivity when their capabilities are augmented with human skills.
In the Collaborative Intelligence Playground, we are going to foresee two major developments.
- Humans Empowering Machines
- Machines Assisting Humans
Though groundbreaking innovations have happened in the arena of AI and Generative AI, AI tools require human intervention to receive training, get feedback, comprehend their behavior to the stakeholders, and to ensure the sustainability of such AI models by continuously monitoring their behavior to ensure Responsible AI.
On the other hand, Human capabilities get a boost by relying on AI tools as they can amplify analytical and decision-making capabilities, relieve human resources from mundane tasks like answering generic customer queries, and act as extensions by doing heavy lifting in manufacturing setups thus providing ‘super-human’ traits to the normal physical capabilities of humans.
Humans Empowering Machines
Training Machines:
Machine Learning algorithms require training to accomplish their designated tasks. For instance, Generative AI text, music, and image generation tools need to be trained on huge data sets, Healthcare apps need training on detecting medical conditions and diseases, and financial recommendation engines also need extensive training to help in decision-making.
Fostering Transparency:
Often the AI behavior requires explanation to be shared with the end users as they must be aware of why AI took a particular decision or made a particular decision so that the complete system is transparent. Writing such explainers is the key responsibility of humans.
Ensuring Responsible AI Capabilities:
Human intervention is required to build sustainable AI systems that adhere to the responsible AI guidelines. It is the responsibility of organizations to produce harmless AI systems.
Machines Assisting Humans
Collaborative Intelligence Engines to Augment Cognitive Capabilities:
Though the human brain is considered as the epitome of intelligence, human experts need AI assistance to speed up decision making process by analyzing a huge amount of data.
For instance, a music composer can rely on AI systems to fetch ideas that enhance the imagination of an exceptional music composer so that an entirely new piece of music can be envisioned. An AI system can churn out a variety of designs that match those criteria, often igniting ideas that the composer might not have initially thought of.
Human-Machine Collaboration Enhances Quality of Customer Interactions:
Collaborative Intelligence redefines the way interactions happen with customers. Organizations can offload all the routine customer-service queries to AI chatbots and ensure that expert customer executives can focus on handling complex customer queries and troubleshooting. A plethora of AI-driven chatbots are available today like Microsoft Copilot, Apple’s Siri, etc.
Collaborative Intelligence Help Personify Human Capabilities
AI tools like Siri and Copilot are basically digital entities that do not have physical existence like robots but recent developments in the collaborative intelligence arena have popularized cobots. The Cobots are robots with intelligence embodied that augments a human worker. These AI systems can do a lot of heavy lifting in manufacturing or factory settings with ‘personified’ human intelligence which was not the case with old-school mechanical robots.
Future of Work: How Collaborative Intelligence Reshape Businesses
Organizations and leaders should be clear on which aspects of their operations need to be redefined before embracing AI-driven collaborative intelligence engines in their systems.
Organizations should decide on the challenges they face with respect to flexibility, speed, scalability, decision-making, or personalization of their solutions or operations.
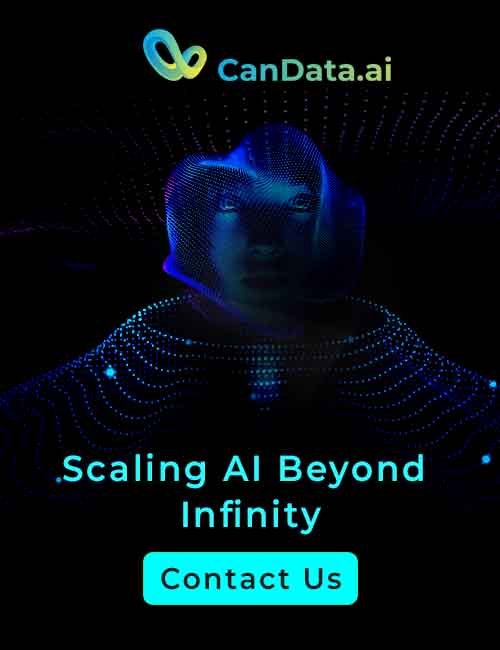
For instance, if you are in the automobile industry and your operational team has flexibility issues in customizing cars that are in high demand, you can switch over to collaborative-intelligence driven human-machine teams where human operators can control cobots by giving precise instructions through tablets which were lacking in earlier mechanical robots.
Similarly, if you are a financial institution like a bank and should make swift decisions (Speed) in fraudulent transaction detection then you must embrace collaborative-intelligence-based AI solutions where AI tools can analyze millions of transactions quickly and Human experts can entirely focus on identifying false positives.
Next, consider your organizational requirement is to Hire on a rapid scale (Scalability) and segregate the new recruits based on their strengths so that they are offered suitable positions in the organization enhancing the overall productivity. Collaborative Intelligence engines assist HR managers in scaling up the prototype and recruiting in massive numbers.
Precision in decision-making is one of the major advantages AI-driven systems offer. By providing employees with the right kind of information and guidance, AI systems improve the quality of decision-making at your organization.
Personalization is the hallmark of best marketing campaigns. AI tools can help your marketing teams deliver precisely personalized offerings to your potential and existing customers. Marketing managers who collaborate with AI tools always have an edge over the competition in reaching the target audience accurately.
In a nutshell, Collaborative Intelligence Engines enable organizations to decode unknowns into knowns in two steps:
- Human Assisting Machines where human resources enable machines to decipher the unknowns by offering explanations and responsible AI solutions.
- Machines Assisting Humans in decoding unknowns to knowns and enable them to realize robust and repeatable solutions.
Key Benefits of Collaborative Intelligence
Here are the salient points listing the benefits of Collaborative Intelligence.
Foolproof inspection for analysis – In a bank or financial institution, AI agents can quickly analyze millions of transactions while Human experts can analyze any false positives that have crept in.
Improve Performance and Efficiency – AI agents deliver accurate outcomes without any fatigue. At the same time, humans can focus on other high-priority tasks which require high-level cognition.
Cost-effectiveness – By embracing human-AI collaboration, organizations can drastically reduce the cost-per-contact by 80% during customer service interactions.
Enhance customer experience – With collaborative intelligence, organizations can improve customer experience by predicting customer preferences and offering personalized experiences. AI agents can help analyze data patterns, and human agents can focus on understanding your customers’/ prospects’ requirements and invest more time in facilitating them.
Customization of AI agents is Advantageous – With high-level of cognition, Human agents have innate qualities to be brand ambassadors. This quality can be imposed on AI gents through systematic training so that they can act as brand ambassadors in future conversations by adhering to the company’s requirements.
Scalability is the Power of AI agents – AI agents offer the power to scale customer service activities during peak hours. AI agents in manufacturing setup can also help in boosting production activity.
Challenges Ahead for Collaborative Intelligence Engine Designers
Here are the unique challenges that can occur during the design of Collaborative Intelligence Engines:
- Balancing Act between Trust and User Control: As AI systems gain more sophistication human experts can become circumspect about their behavior. Having the right balance between personalization and user control is essential. Helping humans understand how their data is used and providing options to opt out of specific features can build trust.
- Aligning AI and Human Goals:
Though both human agents and AI agents have the motto of enhancing customer experience their strengths differ. While AI agents work on delivering services quickly human agent’s goals will be to build rapport with customers. Thus, both should align their goals seamlessly.
- Evolving Customer Expectations: Customer expectations rapidly change, and it is a challenging ask for Collaborative Intelligence Engines to keep pace with the changing needs. This leads to continuous learning requirements through new data and interactions.
- Ethical Considerations: Beyond privacy concerns, ethical considerations regarding AI’s role in customer service need to be addressed. For example, how
will AI handle emotionally charged situations or complex ethical dilemmas? Developing clear guidelines for AI behavior and ensuring human oversight can help mitigate potential ethical issues.
- Measuring Success: Measuring the efficiency of a collaborative intelligence engine can be tricky. While traditional metrics like customer satisfaction scores are important, it’s also valuable to consider metrics that reflect the effectiveness of human-AI collaboration, such as agent sentiment and efficiency gains.
In addition, here are the generic challenges encountered while building AI systems
- Ensuring High-Quality Data and Integration: These engines rely heavily on data from various sources – customer interactions, purchase history, and social media. Integrating this data seamlessly and ensuring its accuracy is crucial. Inconsistent or incomplete data can lead to flawed predictions and hinder the effectiveness of the system.
- Privacy Concerns: Using vast amounts of customer data raises privacy concerns. Balancing the need for personalization with data privacy regulations requires careful consideration.
- Explainability and Bias: The solutions and recommendations given by AI models are often complex. It is difficult to understand how they arrive at decisions. Providing explanations for a machine’s behavior can prove complicated when dealing with customer issues. In addition, biases occurring due to flawed training data can be reflected in the AI’s behavior, resulting in poor outcomes.
Salient Collaborative Intelligence Projects
Here is a list of salient Collaborative Intelligence projects offered by major players like Google and Microsoft:
Project Name | Description |
Teachable AI Experience (Microsoft) | A project to rethink the way AI innovators interact and collaborate to build truly inclusive and human centric experiences |
Google AI Platforms (Google) | This platform enables AI practitioners and researchers to interact, collaborate, and build, train, and deploy Machine Learning models. |
Project Alexandria (Microsoft) | This project is aimed for automatically extracting business knowledge into one consistent knowledge base. This project enables human resources to collaborate effectively with organizational knowledge. |
TensorFlow Playground (Google) | This interactive tool enables Machine Learning enthusiasts to learn from easy-to-use visual interface. |
TrueSkill (Microsoft) | This collaborative tool is employed to assess and record the skills of computer gamers. |
Kaggle Competition (Google) | Hosts competitions amongst Machine Learning practitioners to assess their skills. |

We can conclude this blog with a broader classification of Collaborative Intelligence provided by the Frontiers of Collaborative Intelligence Systems research paper. The Collaborative AI is broadly classified into three levels: micro, meso, and macro. The Micro level of collaboration is defined by swarm intelligence collaborative methods about individual collaboration and decision variables collaboration. The meso-level collaboration highlights multi-task collaboration and multi-party collaboration. And finally, the macro-level collaboration is focused on delivering intelligent collaborative systems, such as terrestrial-satellite collaboration, space-air-ground collaboration, space-air-ground-air collaboration, vehicle-road-cloud collaboration, and end-edge-cloud collaboration.
In a Nutshell
Collaborative Intelligence Engines represent the future of human-AI partnerships, where both human creativity and AI’s efficiency are harnessed. This synergy enhances productivity, optimizes decision-making, and elevates customer experiences. By embracing these engines, organizations can achieve scalable, cost-effective, and innovative solutions, positioning themselves at the forefront of technological advancement.